Technology is infamous for falling into a cycle of hype, with its ups and downs of inflated expectations and disappointment – AI-based drug discovery is no exception. In this article, Aaron Daugherty, Vice President of Discovery at Aria Pharmaceuticals, discusses how industry can use AI to transform research.
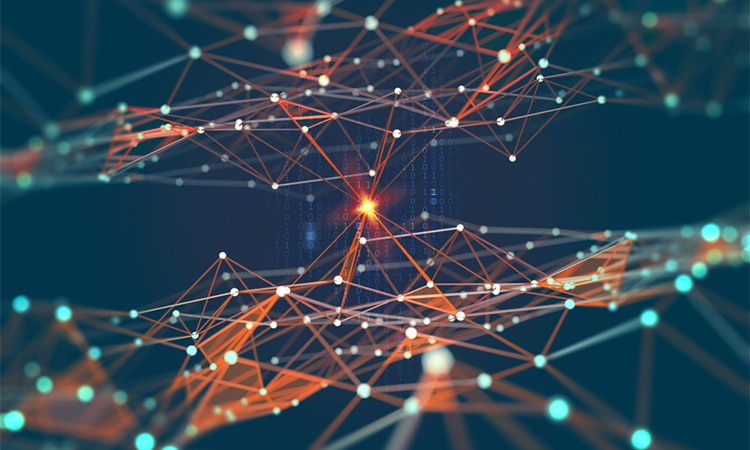
For more than a decade, the promise of artificial intelligence (AI) has been overstated by the idea that it will revolutionize pharmaceutical research in ways we never thought possible. This hype, as seen in headlines and at conferences around the world, has created unrealistic expectations for AI, which is capable of single-handedly solving complex biological problems and delivering breakthrough treatments to unthinkable speed. Obviously, this has not been the case so far.
McKinsey & Company has identified nearly 300 companies operating in AI-based drug discovery, but double notes that only a small percentage have built a pipeline of assets moving into preclinical research. Furthermore, most of the even smaller fraction of companies with clinical research candidates have licensed these assets or developed them using traditional discovery approaches. This data tells us that the results delivered by many AI-powered drug discovery companies to date have not lived up to initial expectations.
Recently, however, the conversation about the role of AI in drug discovery has begun to shift into a more productive and practical examination of its true potential as a tool to support scientists through the discovery process. of drugs. Hopefully this collapse of hype into a realistic new reality doesn’t dampen further investment or interest, as significant progress is still being made – just not at the level heralded over the past decade. In fact, we are entering an exciting and promising new phase of AI in drug discovery; highlighted by a clearer understanding of the true value of AI in this data-heavy industry: for problem solving.
AI as a complement, not a substitute
The first step is to not let a perceived lack of progress dictate the future application of AI in drug discovery. The focus will continue to be on using AI to help alleviate many of the complexities of drug discovery. However, it must be recognized that AI has limitations and must therefore be integrated with care. I would argue that a good integration uses AI as a set of tools that supports and accelerates scientific discovery work rather than replacing it. Essentially, AI can improve drug discovery, but only with proper application.
Trends in 2023
One trend that I have noted, and alluded to before, is a move toward integration into the functions of existing drug discovery teams. More and more biopharmaceutical companies understand the value of AI and as such there is greater integration between biomedical scientists and data scientists. Much of the initial hype was because too many companies said they could use AI to find drugs faster and better, but failed to recognize that high-quality labs and clinical science are a must. prerequisite for ultimate success.
Proprietary data, software and technology are all wonderful talking points, but they are useless if not informed and guided by the expertise of pharmaceutical scientists. Technology and AI should never aim to replace researchers. It should aim to make them super-powerful. At the same time, technology and data experts need an equal place at the table alongside biologists, chemists, clinicians and others to make an impact. The future is one of integration between all the disciplines necessary to make effective medicine.
The second trend is a growing understanding of the extreme complexity of some chronic diseases and a recognition that AI will be needed to untangle these biological complexities. As we collect increasing amounts of knowledge about disease, making sense of the data becomes increasingly difficult.
I would argue that a good integration uses AI as a set of tools that supports and accelerates scientific discovery work rather than replacing it”
A fundamental problem with traditional drug discovery is that it necessarily uses a reductionist approach – searching for potential treatments with only a limited view of disease pathology. Scientists will screen compounds against one or two aspects of a complex disease, whether it is the activity of a single protein target or the modification of a single phenotype. However, as our understanding of disease becomes more complex and interconnected, this traditional approach to finding treatments is rapidly becoming limited.
This is where AI has value to break through. AI allows us to interrogate potential treatments against completely unconnected multimodal data at the same time, giving us a more complete picture of disease biology and, therefore, a better understanding of how a given treatment will affect the disease. Rather than facing a decline in pharmaceutical breakthroughs alongside growing disease complexity, these new tools can help us continue to innovate and find new treatments. Moreover, as more data becomes available, the complexity of the disease, when coupled with the capabilities of AI, becomes an asset in finding better, safer and more effective drugs.
Finally, not so much a trend as an inevitable next step, is that drugs discovered by integrated medical and data science teams will begin to make their way into clinical research in critical mass. A few things interest me as this happens: the first is whether these candidates will see a higher success rate than those uncovered through traditional methods. The second is whether a given candidate produces a breakthrough in the way we treat disease, which would be a mechanism of action (MOA) never considered before or an entirely new approach. The value of AI was positioned against the machines’ speed, efficiency and ability to identify signals that would otherwise be missed by traditional screening methods. In other words, if the AI discovers a “me too” candidate who ultimately succeeds, what value beyond the obvious have we achieved?
Deploying technology to make the traditional approach to drug discovery more efficient and faster is not inherently bad, but it does not aim to solve the real problem, which is the complexity of human disease. AI, at its best, processes data with precision and speed unthinkable at scale. The growing amount of data we have on thousands of diseases is insurmountable with current approaches, but it is essential for finding breakthroughs.
No one can predict the future of clinical work ahead of us, but I firmly believe there will be a line drawn in the sand. Companies that focus exclusively on building AI software and using proprietary datasets will see some success, but not at the bar set in the first whirlwind of hype. Companies looking to to integrate AI in the pharmaceutical research process targeting this looming problem of growing disease complexity and masses of data will innovate harder and contribute to rethinking and rethinking pharmaceutical R&D entirely.
Technology and AI should never aim to replace researchers. It should aim to make them superpowered”
It should be noted that pharmaceutical R&D is risky. However, AI offers significant potential to increase our success rates by focusing on new candidates that have the best chance of safety and efficacy in humans.
All metrics point to 2023 as the year we move from hype and unrealistic expectations to integrating AI into pharmaceutical discovery that focuses on the problems we’re trying to solve with realistic expectations. We are heading towards a future where the true value of AI will impact productivity in the area of difficult-to-treat and complex diseases. Some companies may suffer from not delivering on the overpromised hype, but the strongest players will help drive important new innovations and discoveries that benefit patients.
Aaron C Daugherty, Vice President of Aria Pharmaceuticals, helped build Aria’s drug discovery platform and leads the efforts of the Discovery Science team to uncover potential treatments for a wide range of diseases. Aaron received his PhD in Genetics from Stanford University, USA. Prior to his time at Stanford, Aaron was a Fulbright Scholar and earned his BSc in Biology from the University of Richmond, USA.